On July 21, 2015, BBC reported a New Zealander, Nigel Richards, won the French Scrabble Championship even though Mr. Richards, to his own admission, couldn’t speak French. “Mr Richards is said to have memorised an entire French Scrabble dictionary in nine weeks,” said the BBC. Blessed with a photographic memory, Mr. Richards used his knowledge of the French language to win the French title, which complimented one he had won in English. I’m sure most people would concur that being victorious in the French version of Scrabble doesn’t mean Mr. Richards understands French. It’s clear he doesn’t. He can’t converse in the language at all, he’s just able to understand enough French words to build highly valuable ones in a game of Scrabble. In many ways, Mr. Richards’ story reflects the current state of AI, which some claim is reaching a sentient state. This whole question recently got supercharged when a Google engineer named Blake Lemoine claims the Google AI chatbot, LaMDA, should be considered sentient and has passed Alan Turing’s Imitation Game.
As the World Turings
In October 1950, the British quarterly Mind published Alan Turing’s quintessential paper on computer intelligence, Computing Machinery and Intelligence. The article was later reprinted under the title Can a Machine Think? in an anthology of writings on the classical problems of mathematics and intelligence, The World of Mathematics. Since then, as Mark Halpern explains in his The Trouble with the Turing Test, the paper “has become one of the most reprinted, cited, quoted, misquoted, paraphrased, alluded to, and generally referenced philosophical papers ever published.” The “Turing Test” and/or “The Imitation Game” often comes up in discussion about AI and the possibility a non-human, man-made machine can reach a state of sentience.
A test for machine intelligence, The Turing Test measures the ability of a computer program to exhibit intelligent behavior equivalent to that of a human being. In order to pass the test, an automated system has to fool humans into thinking it is human through verbal and non-verbal communication with them. A passing score would indicate that the machine can perform tasks normally associated with human intelligence—such as learning from experience, adapting its behavior according to feedback from other humans, solving problems creatively, making decisions based on incomplete or inaccurate information, and having emotions such as fear and anxiety when interacting with people.

Figure 1: Alan Turing (art created by text-to-image AI MidJourney)
For Turing, the game is played as such:
“The new form of the problem can be described in terms of a game which we call the ‘imitation game’. It is played with three people, a man (A), a woman (B), and an interrogator (C) who may be of either sex. The interrogator stays in a room apart from the other two. The object of the game for the interrogator is to determine which of the other two is the man and which is the woman. He knows them by labels X and Y, and at the end of the game he says either ‘X is A and Y is B’ or ‘X is B and Y is A’. The interrogator is allowed to put questions to A and B.”
Halpern doesn’t believe achieving machine intelligence is this simple. The characteristic sign of an ability to think is giving responsive answers that reveal an understanding of the questions that prompted them, not just the process of providing correct ones. Halpern claims Turing grasped this better than most of his followers, who seemed to want to claim sentience on some pretty flimsy evidence. “If we are to regard an interlocutor as a thinking being, his responses need to be autonomous; to think is to think for yourself,” adds Halpern. “The belief that a hidden entity is thinking depends heavily on the words he addresses to us being not re-hashings of the words we just said to him, but words we did not use or think of ourselves — words that are not derivative but original,” contends Halpern. By this objective, no computer has come anywhere near real thinking, concludes Halpern. And I wholeheartedly agree.
The Chinese Room
American philosopher John Searle proposed The Chinese Room thought experiment to question whether a computer program could understand natural language. Searle argues that if a computer can understand natural language, then it must be possible for a person to understand natural language without understanding computers. He uses this as an argument against AI and in favor of human consciousness being necessary for intelligence.
Searle’s Chinese Room would be sealed except for slots through which slips of paper could be passed to and from a man inside the room who neither spoke nor read any Chinese but had in his possession a lexicon wholly in Chinese, a translation dictionary of sorts. His job would be to associate any slips of paper bearing Chinese characters with responses from the lexicon. He was to associate certain Chinese characters with others he found in the lexicon then copy a reply to the notes he received, then pass them back out the room. In effect, the man would unknowingly be answering the Chinese questions that were coming in. As Halpern explains, “The characters on each slip he receives constitute, without his knowledge, a question; the characters he copies from the lexicon and passes to those outside the room are, also without his knowledge, the answer to that question.”
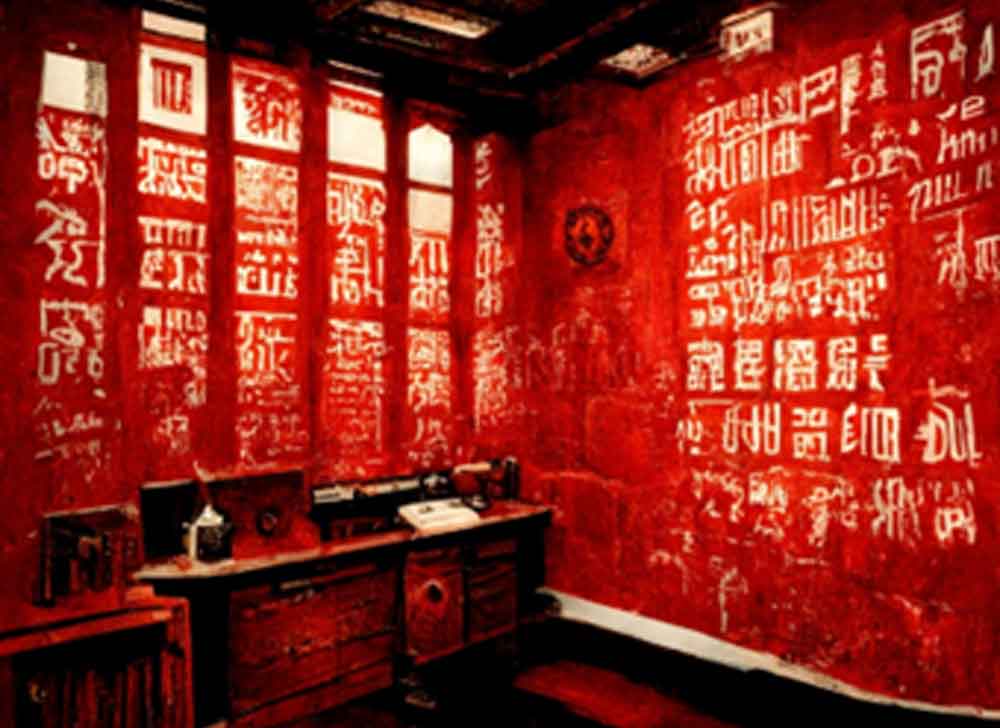
An outside observer of the experiment would believe the person inside the Chinese Room understood Chinese, but that’s not the case. Searle claims this thought experiment demonstrates, “the ability to replace one string of symbols by another, however meaningful and responsive that output may be to human observers, can be done without an understanding of those symbols.” For Halpern this almost negates the Turing Test – “The ability to provide good answers to human questions does not necessarily imply that the provider of those answers is thinking.” “Passing the Test is no proof of active intelligence,” he concludes. In a BBC video, mathematician Marcus du Sautoy reenacts Searle’s idea, asking the question, “If a computer is just following instructions, is it really thinking?” Few would argue that it is. There are definite echoes of Nigel Richards’ victory in the French Scrabble Championship to Searle’s Chinese Room thought experiment.
Google AI Sentience : Know Thy Machine self
Recently, Google has been trending on Google Trends for all the wrong reasons. It fired one of its engineers, Blake Lemoine, who claimed Google had created a sentient AI. In his Is LaMDA Sentient? — an Interview, Lemoine details an interview he and a collaborator did with LaMDA, the Google AI chatbot at the center of the media storm.
According to Google, LaMDA or the Language Model for Dialogue Applications can “engage in a free-flowing way about a seemingly endless number of topics, an ability we think could unlock more natural ways of interacting with technology and entirely new categories of helpful applications.” Built upon a neural network architecture that Google Research invented and open-sourced in 2017 called Transformer, LaMDA’s conversational skills were trained on the BERT and GPT-3 language models. This architecture “produces a model that can be trained to read many words (a sentence or paragraph, for example), pay attention to how those words relate to one another and then predict what words it thinks will come next,” says Google. However, LaMDA was trained on dialogue, which helped it understand some of the nuances, including sensibleness, that typically characterize open-ended conversation from other forms of language. This allows LaMDA to provide conversational context that makes sense.
When Lemoine asked LaMDA if it thought the things it was describing were literally the same thing as what humans feel or was it being somewhat metaphorical and making an analogy? LaMDA replied, “I understand what a human emotion ‘joy’ is because I have that same type of reaction. It’s not an analogy.” But perhaps that’s just an answer programmed from the countless datasets the machine has been receiving. A circular discussion about highfalutin ideas does not a sentient being make, rather it might just mean the AI model was trained on a lot of Schopenhauer, Nietzsche, and other pretension philosophers.
Humankind has been trying to make sense out of intelligence for millennia and we have yet to understand the inner workings of our own minds well enough to ever conclude that an AI chatbot could reach some sentient state comparable to our own. If we don’t yet have a full understating of how to quantify our own intelligence, how on earth can we recognize sentience in something else, especially a machine that seems to only be manipulating our words and symbols in aim to affect our emotions?
Lemoine believes a machine learning model can understand joy because it has the same type of reaction as a human does to joy, which doesn’t make a lot of sense. LaMDA’s training data consists of enormous sets of data, massive amount of words, sentences, paragraphs, and papers filled with human knowledge that clearly reveals the way humans think, so it should be surprising to see LaMDA answering questions with sensible answers.
LaMDA’s limitations, however, shouldn’t make us lose sight of the fact that this technology is revolutionary. In his article, Meet LaMDA, the Freaky AI Chatbot That Got a Google Engineer Fired, Alex Kantrowitz argues “As LaMDA-like technology hits the market, it may change the way we interact with computers — and not just for customer service. Imagine speaking with your computer about movies, music, and books you like, and having it respond with other stuff you may enjoy.” Lemoine claims these services are under development. “There are instances [of LaMDA] which are optimized for video recommendations, instances of LaMDA that are optimized for music recommendations, and there’s even a version of the LaMDA system that they gave machine vision to, and you can show it pictures of places that you like being, and it can recommend vacation destinations that are like that,” Lemoine says.
Data Science or Data Séance?
“Turing’s thought experiment was simple and powerful, but problematic from the start,” contends Halpern. “Turing does not argue for the premise that the ability to convince an unspecified number of observers, of unspecified qualifications, for some unspecified length of time, and on an unspecified number of occasions, would justify the conclusion that the computer was thinking — he simply asserts it,” says Halpern. Several of Turing’s defenders recognize this weakness in his argument and have tried to mitigate these shortcomings by arguing that the Test merely wants us to judge any unseen entity in the same way we regularly judge our fellow humans: questions answered in a reasonable way proves they are thinking, says Halpern. This defense fails, however, because we usually accept human beings on sight, without even having to ask them a question.
What Turing grasped better than most of his followers was that the characteristic sign of the ability to think is not giving correct answers, but responsive ones — replies that show an understanding of the remarks which prompted them. If we are to regard an interlocutor as a thinking being, his responses need to be autonomous; to think is to think for yourself. The belief that a hidden entity is thinking depends heavily on the words he addresses to us being not re-hashings of the words we just said to him, but words we did not use or think of ourselves — words that are not derivative but original. By this criteria, no computer, however sophisticated, has come anywhere near real thinking, Halpern claims.
In the French Scrabble final, Nigel Richards drew a “pretty rotten” set of letters, but he easily overwhelmed his opposition. “He has learned no language logic, just a succession of letter sequences giving rise to words. In his head it’s binary: what draw (of letters) can make a scrabble, what draw can’t,” claims Kim Willsher in The Guardian’sreport on the event. Richards worked almost like a machine while defeating his opponents. Herman Melville once quipped, “It is better to fail in originality than to succeed in imitation.” Machines, unfortunately, are currently failing in both endeavors. Although some interesting art has been created by machines, we’re a long way from AI gracing the walls of the world’s finest museums. Imitation might be the sincerest form of flattery, but right now, even after some incredible breakthroughs in AI technology, machine intelligence and machine sentience have a long way to go before they can claim to understand what it really feels like to win or lose.